Sparse Multimodal Vision Transformer for Weakly Supervised Semantic Segmentation
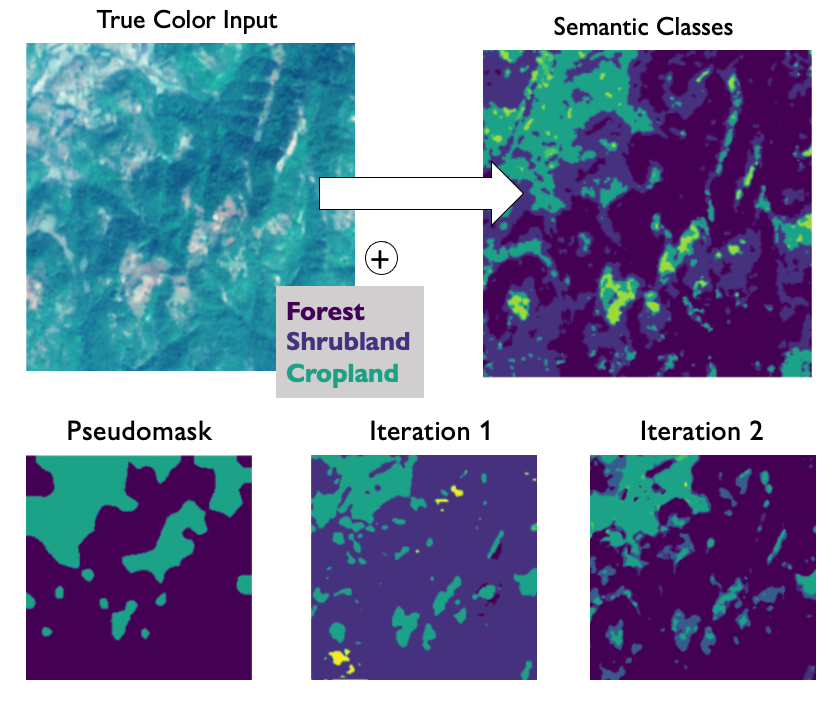
Vision Transformers have demonstrated their versatility and effectiveness in tackling complex computer vision tasks such as land cover segmentation in remote sensing applications. While they perform comparably or even better than Convolutional Neural Networks (CNNs), Transformers typically require larger datasets with detailed annotations, such as pixel-level labels for land cover segmentation. To address this limitation, we propose a weakly-supervised vision Transformer that utilizes image-level labels to learn semantic segmentation and reduce the need for extensive human annotation. This is achieved by modifying the architecture of the vision Transformer, incorporating gating units in each attention head to enforce sparsity during training and retain only the most meaningful heads. After training on image-level labels, we extract the representations from the remaining heads, cluster them, and construct pseudomasks serving as labels for training a segmentation model. Furthermore, we can refine our predictions through post-processing the pseudomasks and iteratively training a segmentation model with high fidelity. Our code is available at github.com/HSG-AIML/sparse-vit-wsss. Evaluation on the DFC2020 dataset demonstrates that our method not only produces high-quality segmentation masks using image-level labels but also performs comparably to fully-supervised training relying on pixel-level labels. Our results also indicate that our method can accomplish weakly-supervised semantic segmentation even with small-scale datasets.
Our weakly-supervised semantic segmentation approach consists of a pipeline with three stages. In the first stage, we train a Transformer model for multi-label classification. During the training process, we enforce attention sparsity by adding learnable gating units to each head of the Multi-Head Self-Attention (MHSA). In the second stage, we generate pseudomasks by extracting attention maps from the last block of the Transformer, reshaping them and clustering them. Each cluster is assigned a label that will be used to create the corresponding pseudomask. In the final step, we refine the generated pseudomask through multiple stages of supervised training of a segmentation model, utilizing the pseudomasks generated in the previous iteration as supervision.

Our results show that pruning over 70% of the heads in a vision Transformer has minimal impact on performance, highlighting their redundancy. The remaining heads, on the other hand, are meaningful and specialized, enabling their utilization for inferring pseudomasks in land-cover mapping.

Moreover, we are able to improve the generated pseudomasks with a refinement step. This consists of training a segmentation model in a supervised way by using as supervision the masks generated by the previous iteration.

To conclude, our work demonstrates the potential to create pseudomasks for segmentation using weak supervision (relying only on image-level labels as supervision) by leveraging representations learned by vision Transformers.
The results of this study have been presented at the CVPR 2023 EarthVision workshop in Vancouver, Canada.